New publication in Physical Review Letters
Growth processes allow us to better understand many of the complex properties of networks, which are by nature dynamic objects. We can go further and assume a certain growth model did generate the structure, allowing us to perform Bayesian inference on the past states of the network. As it has been shown in a previous study from Dynamica, temporal reconstruction is, however, a very difficult task.
The paper entitled Inference, Model Selection, and the Combinatorics of Growing Trees, introduces a glimpse of hope for rigorous temporal reconstruction of networks. In this project, led by George T. Cantwell, current Dynamica member Guillaume St-Onge, and alumni Jean-Gabriel Young, the authors derive exact and efficient inference methods for growing trees and demonstrate them in a series of applications: network interpolation, history reconstruction, model fitting, and model selection.
Links to the full text and preprints are available on the publication page.
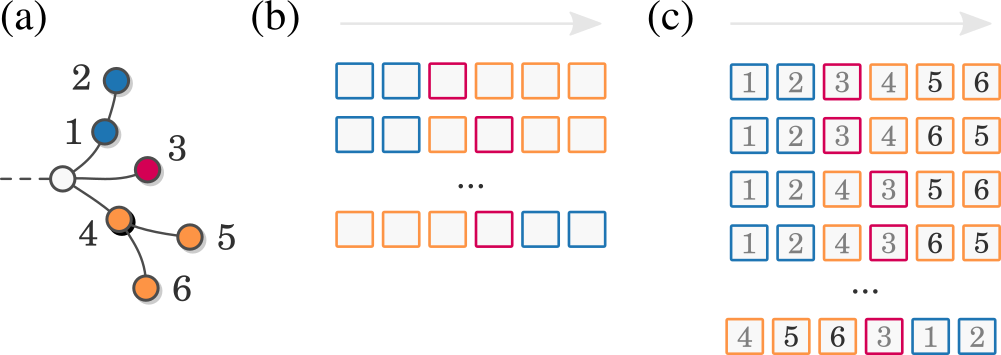